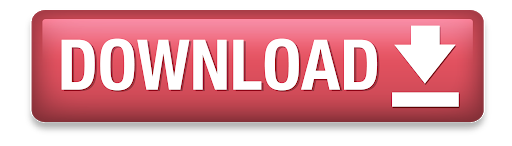
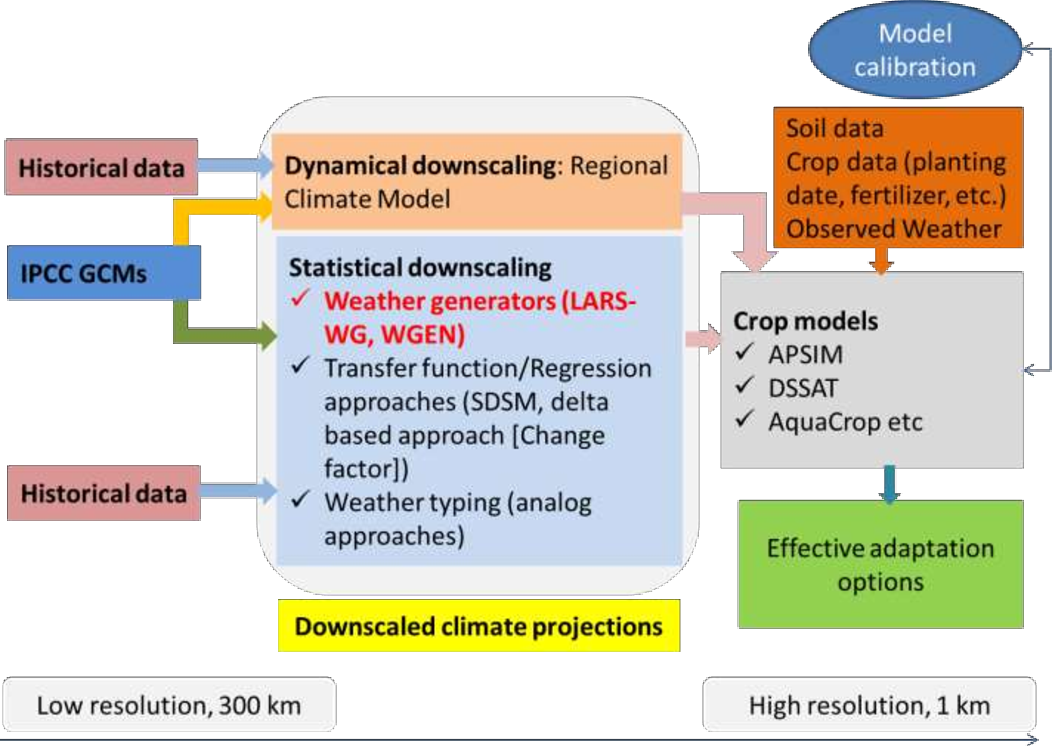
Data on two primary outcomes (abstinence and time to dropout from treatment) were collected in a trial evaluating the effectiveness of a behavioural intervention for substance abuse and data on four primary outcomes were collected in a trial evaluating a multidisciplinary intervention in patients following a stroke. For example, a third of neurology and psychiatry trials use multiple primary outcomes. The use of multiple primary outcomes (or ‘endpoints’) is becoming increasingly common in RCTs. Multiple primary outcomes may be specified in a randomised controlled trial (RCT) when it is not possible to use a single outcome to fully characterise the effect of an intervention on a disease process.
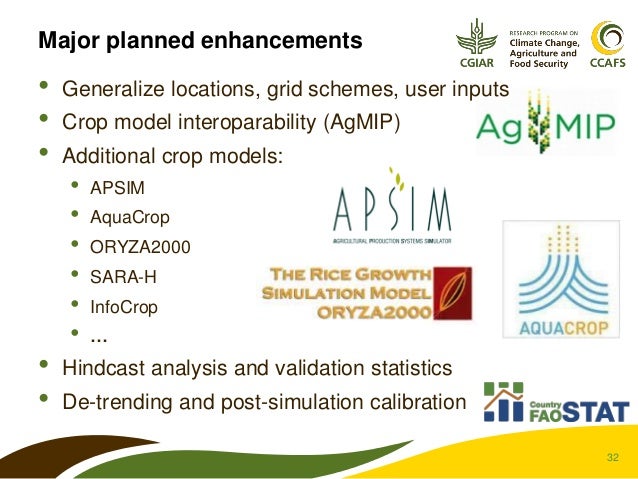
The sample size requirement to achieve the desired disjunctive power may be smaller than that required to achieve the desired marginal power. The choice between whether to specify a disjunctive or marginal power should depend on the clincial objective. However, it removes participants with missing values prior to the analysis resulting in a loss of power when there are missing data.

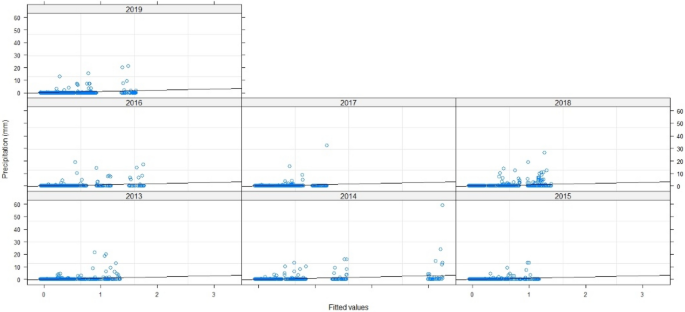
The Stepdown-minP procedure performs well for complete data. The Hochberg and Hommel methods provide power gains for the analysis, albeit small, in comparison to the Bonferroni method. The Bonferroni and Holm methods provide the same disjunctive power. Different simulation scenarios were constructed by varying the number of outcomes, degree of correlation between the outcomes, intervention effect sizes and proportion of missing data. We use simulation studies to investigate the disjunctive power, marginal power and FWER obtained after applying Bonferroni, Holm, Hochberg, Dubey/Armitage-Parmar and Stepdown-minP adjustment methods. We also discuss the implications on the sample size for obtaining 90% disjunctive power and 90% marginal power. We provide practical recommendations on which method may be used to adjust for multiple comparisons in the sample size calculation and the analysis of RCTs with multiple primary outcomes. In the context of multiple outcomes, depending on the clinical objective, the power can be defined as: ‘disjunctive power’, the probability of detecting at least one true intervention effect across all the outcomes or ‘ marginal power’ the probability of finding a true intervention effect on a nominated outcome. It’s also important to consider the power of the trial to detect true intervention effects. A popular approach to do this is to adjust the p-values corresponding to each statistical test used to investigate the intervention effects by using the Bonferroni correction. When analysing multiple outcomes it’s important to control the family wise error rate (FWER). Multiple primary outcomes may be specified in randomised controlled trials (RCTs).
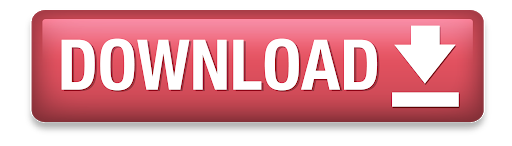